The ROC team will host a talk by Keun-Woo Lim, Associate Professor at Telecom Paris, France. Following his presentation, there will be talks by PhD students.
When: April 11, 2025 at 2 pm to 4 pm.
Where: room 33.1.27C, 2 Rue Conté, CNAM, Paris, France.
Speaker Keun-Woo Lim , Telecom Paris
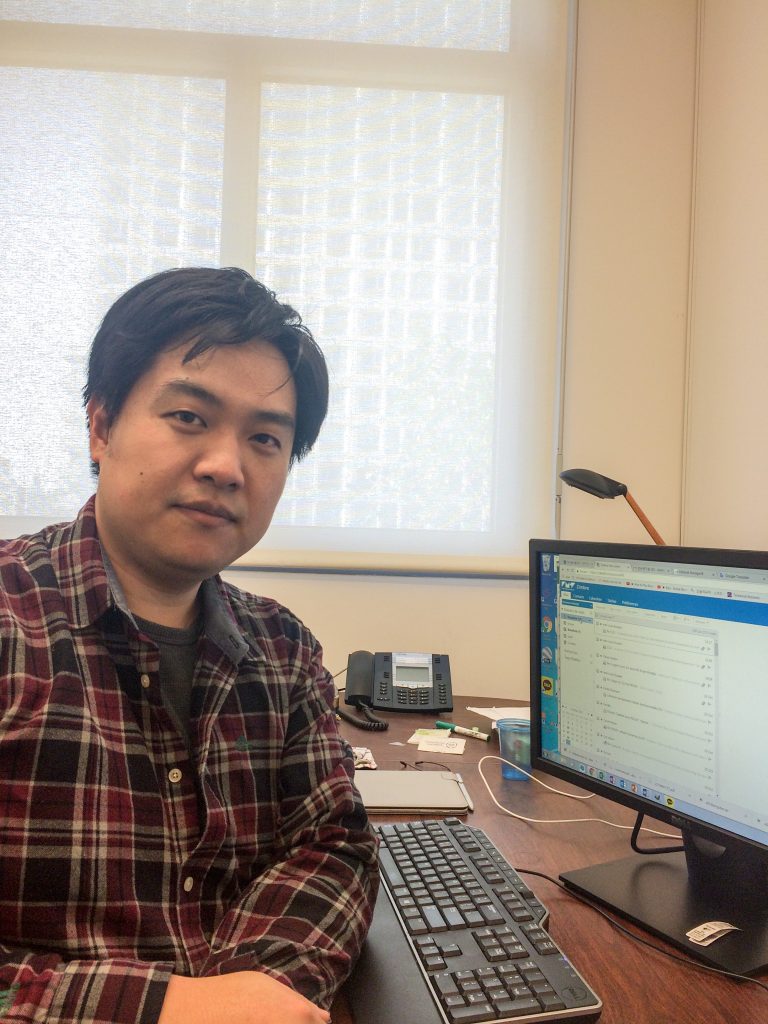
Title: Energy-efficient real-time representation of mobile IoT entities in Digital Twin
Abstract: This work studies the relation between real-time representation accuracy and energy efficiency of mobile IoT entities in Digital Twins applications, and proposes a machine learning based approach to efficiently select the appropriate parameters. Digital Twins is considered as the next evolution of the current Internet, providing connectivity between physical and virtual entities to perform various tasks and operations. However, graphical real-time representation of mobile entities requires continuous influx of data generated by sensors, which in turn can be a great burden for IoT devices that are energy constrained. This work observes the trade-off between real-time representation of mobile entities in Digital Twins using UWB-based localization, to its energy consumption induced from UWB communication through testbed experiment. We show that through measurement of the dynamicness in the mobility, localization sampling rate can be controlled without impacting the accuracy of visual representation. Then, based on the observations, the paper proposes a simple machine learning approach, using Support Vector Machine (SVM), to reduce energy consumption while maintaining a high-level of representation accuracy. Through empirical studies, our tests show that under specific circumstances, we allow devices to sleep 30 percent more while keeping the relative error rate to under 2 percent.
Speaker June-Pyo Jung, PhD student at Telecom Paris
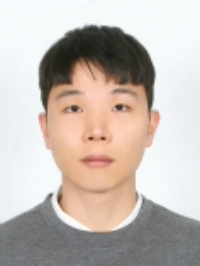
Title: FSTL(Federated Semantic Temporal Learning): Enhancing Local Forecasts and Enabling Global Semantic Predictions from Multivariate IoT Data
Abstract: Federated learning has emerged as a promising paradigm for privacy-preserving machine learning in resource-constrained IoT environments. However, traditional federated learning frameworks often overlook temporal dependencies and semantic correlations among multivariate data, limiting their predictive capabilities, especially when aiming to integrate knowledge from multiple sources for higher-level global predictions. In this paper, we introduce Federated Semantic Temporal Learning (FSTL), a novel federated learning framework explicitly designed to capture semantic states derived from temporal dependencies in multivariate IoT data. FSTL enhances local forecasting accuracy by embedding temporal relationships into meaningful semantic representations at each local device. These semantic states, generated independently from local datasets, are collaboratively aggregated across devices through a federated learning mechanism. This aggregation process enables the synthesis of global semantic states, allowing a new tier of global predictions that leverage combined semantic insights from multiple IoT devices. By explicitly integrating temporal semantics, FSTL achieves comprehensive predictive capabilities while maintaining efficient resource usage and data privacy. This research opens new avenues for leveraging temporal semantics in federated IoT settings, enabling advanced predictive functionalities beyond conventional federated learning frameworks.
Speaker Stefano Taborelli, ROCker PhD student
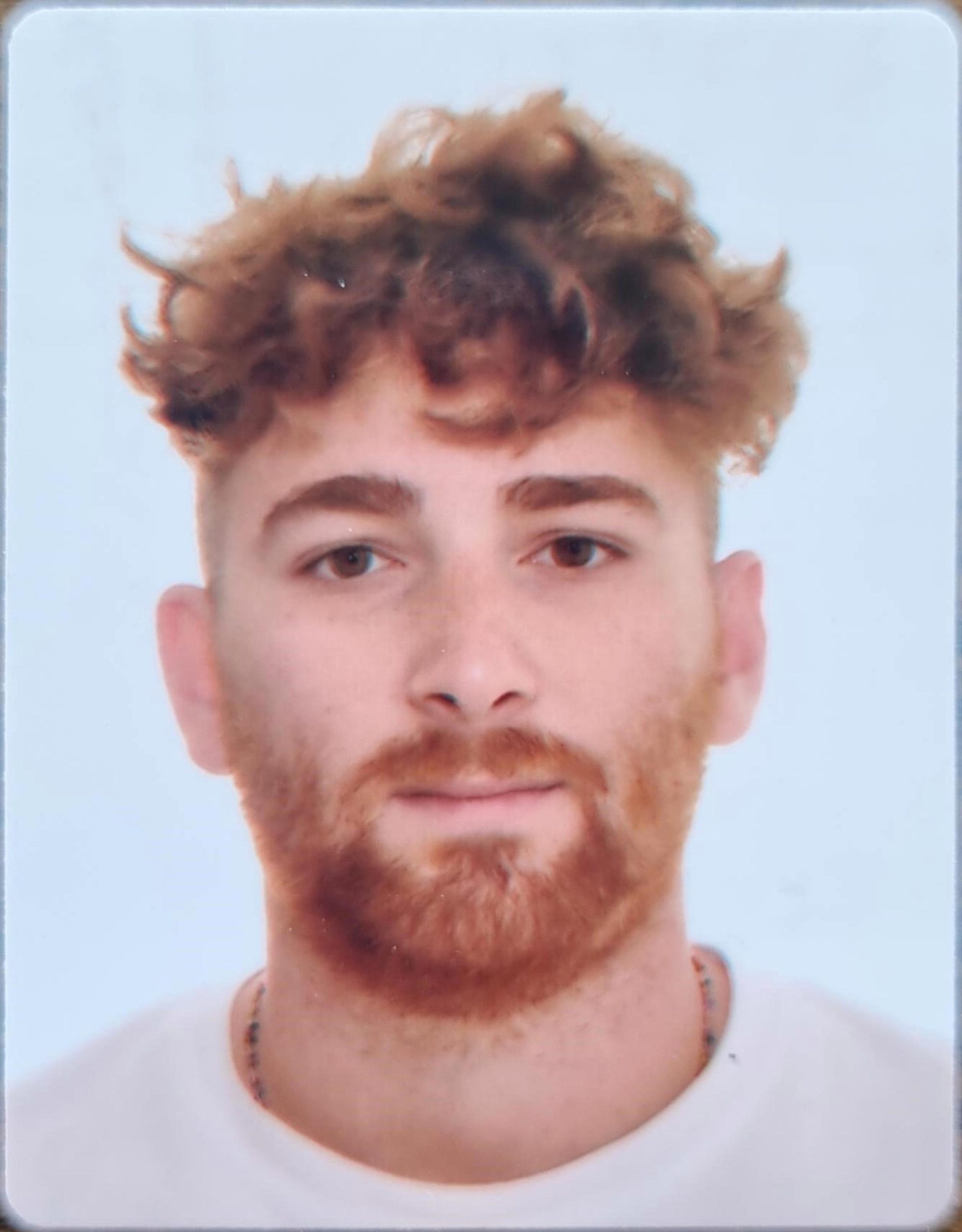
Title: Multi Connectivity in 6G
Abstract: This presentation introduces Stefano Taborelli, a PhD student since February 2025 with a background in Computer Science Engineering from Politecnico di Milano. His professional experience includes roles in application engineering and consulting. Stefano is currently involved in the NexaSphere project, part of Horizon Europe, which focuses on designing a heterogeneous 3D network for future 6G systems. Key objectives include the development of AI-driven orchestration and the integration of terrestrial and non-terrestrial networks to ensure seamless connectivity across different ranges.
His specific contribution lies within Work Package 4 (WP4), aimed at developing multi-connectivity solutions that enable smooth transitions between network types while maximizing resource allocation. The project also includes building a large-scale simulation framework to test proposed solutions. The presentation outlines the motivations for transitioning from 5G to 6G, such as enhanced reliability, throughput, and global coverage, and explores the technical challenges in achieving these goals. Stefano’s future work will focus on the design and implementation of simulation models to study multi-connectivity in realistic scenarios, particularly within TN and NTN contexts.
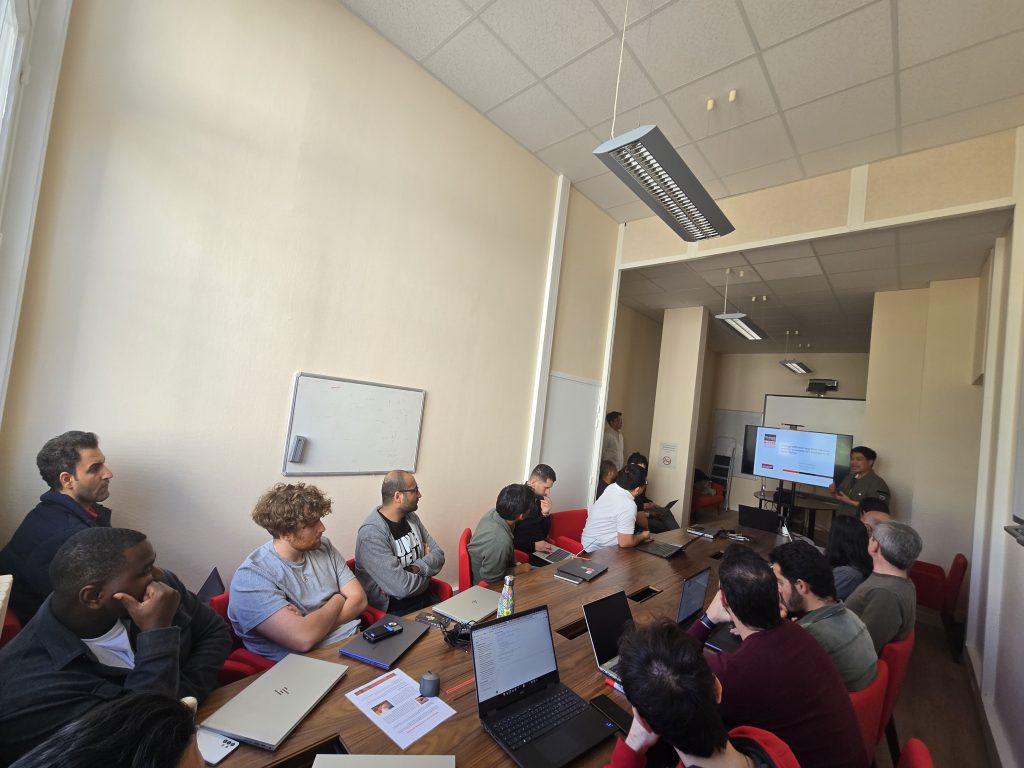
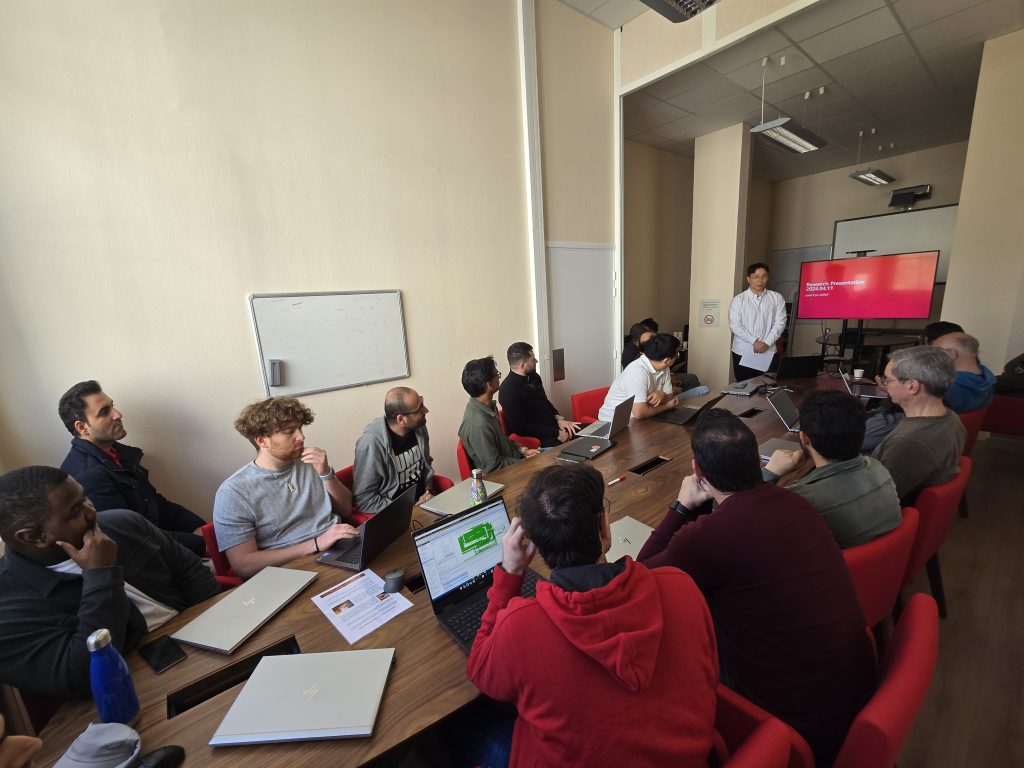
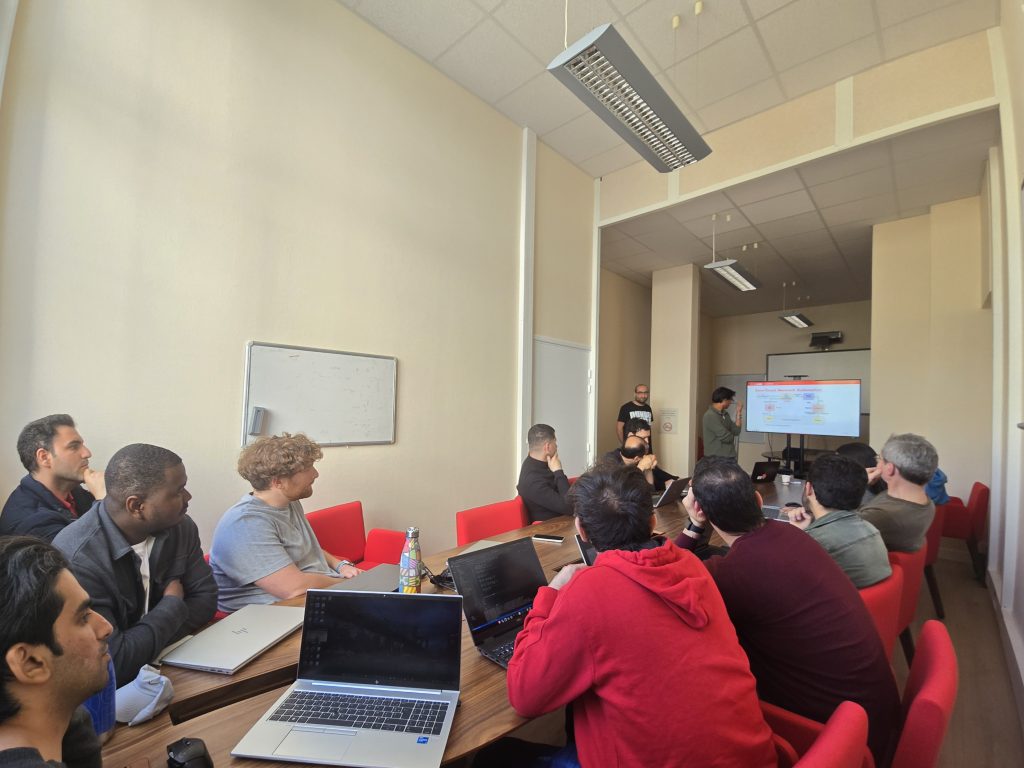